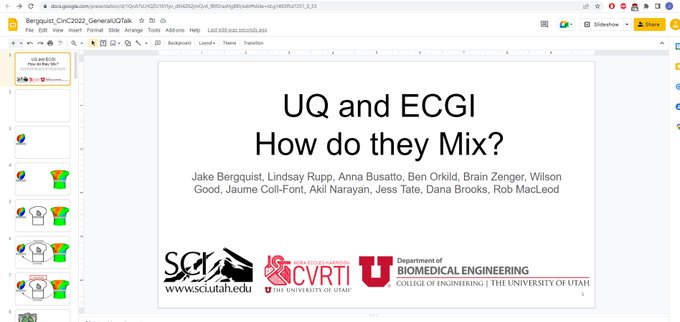
All models are subject to variations and errors associated with their inputs which can arise due to a range of sources such as assumptions made when constructing a model, errors in measuring the inputs to the model, or natural variation in the parameters we use in our models. It is difficult to predict the effects this error and variability in the model inputs can have on the model output. Modern uncertainty quantification techniques employ sophisticated mathematical approaches to allow for the robust evaluation of the effects of these input uncertainties on the model outputs, allowing for exploration of which parameters are important in modelling settings and how variations in these parameters can affect the output. For my presentations, I focused specifically on electrocardiographic imaging as a heart modeling modality.
Electrocardiographic imaging (ECGI) is a noninvasive process for reconstructing the electrical activity of the heart using body surface electrical measurements, similar to those used for the common 12 lead electrocardiogram (ECG). ECGI provides a useful tool to assess the activity of the heart and allow for characterization and diagnosis of heart diseases without the need for costly and dangerous invasive procedures such as catheter mapping. ECGI has already been implemented in several clinical systems for use in diagnostic and preoperative planning in the treatment of cardiac arrhythmias. ECGI uses a model of the heart and the torso, and thus errors in this model such as incorrect placement of the heart in the torso model can affect the accuracy of the ECGI outputs, thereby possibly compromising its clinical utility. The heart’s position varies naturally as a patient breathes or shifts positions, and selecting the location of the heart using medical imaging (such as an MRI scan) can introduce errors as well, however it is not clear how these errors and variations in heart position may affect the ECGI output. To address this, in my talks I described a series of studies we performed to apply uncertainty quantification to investigate the impact of heart position variability on ECGI outputs. From these studies we were not only able to identify which types and amounts of cardiac motion could result in detrimental errors in ECGI solutions but also we were able to formulate methods to account for this variability in heart position to produce an ECGI method that was robust to this variability.
Computing in Cardiology (CinC) is an annual conference that brings together experts across the field of computational cardiology. CinC brings together experts across multiple disciplines to present their work in applying computational techniques to address the detection, diagnosis, treatment, modeliugn, and understanding of cardiovascular diseases. This was my fourth year attending CinC and I can highly recommend this conference to anyone in my field. CinC for me has been an excellent opportunity not only to present my research to a technically minded audience but also to form connections and collaborations with my peers and other research groups from around the world.
This year was no different as I was able to meet with my collaborators from Spain, France, Turkey, Germany, the UK, Slovakia, and Australia, to name just a few. The conference consisted of three days of seminars, talks, and podium presentations with topics ranging from detection of cardiac arrhythmias, modeling the electrical and mechanical functions of the heart, deep learning based approaches for diagnosis of cardiac disease and many more. This year I presented two talks, both relating to uncertainty quantification in the context of cardiac modeling. All presenters (both podium presenters and poster) are invited to submit a 4 page conference paper which is included in a published conference proceedings.
I was drawn to bioengineering for both my love and fascination with biology and medicine, and my passion to build and create things. Outside of my life as a graduate student I make functional ceramics in my home ceramic studio, brew beer in my garage, cook, bike, play music with my friends, and go climbing, backpacking, and hiking with my wife Sonya and our two husky mixes Sage and Willow. I love to learn new things, and share my passions with others. Most recently I have returned from a summer of working in Bordeaux, France with some of my collaborators at the University of Bordeaux, and along the way I picked up just enough French to get by, much to the delight of my Francophone wife. After graduation I am looking forward to seeking a career developing medical technologies and applying computational techniques to better patient care.